
Windows 10 Pro provides all the features of Windows 10 Home + powerful management tools and enterprise grade security.
Windows 10 Pro is built for business
Simple and flexible management - Windows 10 Pro is user-friendly and flexible: connect to Windows 10 business resources with Azure Active Directory.
____________________
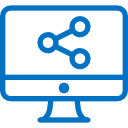
Share and work on documents in real time
_____
Your Office files like Excel, Word, and PowerPoint automatically get stored in the cloud, which acts as a virtual online drive, so you can share and work on documents in real time.
Use your Windows 10 Pro PC anywhere
_____
Start editing a document in the office, keep working on it in the coffee shop on your phone, and finish up on your home PC. You can connect to your business or school Domain or Azure Active Directory to use network files, servers, printer and more. All you need is an internet connection and Remote Desktop.
Cortana is your personal digital assistant
_____
Cortana can provide notifications about appointments and deadlines, suggest documents you may need for an upcoming meeting, and keep your group calendar in sync. Easily create, modify, and open lists with just your voice. And Cortana seamlessly integrates with other Windows apps, like Sticky Notes, Ink reminders, and Microsoft To-Do events.
The multitasking features with Office.
_____
You can arrange your screen however you want. Easily manage multiple Office apps and docs on one screen, and effortlessly snap up to four of them to optimize your workspace. You can also slide the shared edge of snapped apps to wherever you want it, easily resizing both apps in a single motion.Microsoft Windows 10 Pro - an intuitive user experience
An intuitive user experience, with built-in tools such as inking, tablet mode, and touchscreen.
____________________

Start Menu is Back
Windows 10 brings back an old favorite with the Start Menu for ease of access and quick lookup. It also features Cortana for voice assisted control in the start menu.
Windows 10 Continuum
With Windows Continuum you don't have to choose between mobility, touch or desktop peripherals. Continuum allows for devices running Windows 10 to switch between tablet and adding keyboard and mouse for desktop mode.


Windows Autopilot
_____
With Windows Autopilot, start using your new Windows 10 Pro devices quickly, without IT help. Enjoy simple and flexible management.
Windows Ink
_____
Quickly access a Windows Ink Workspace with the click of a digital pen or straight from your taskbar. You can even pull up the Ink Workspace before logging in.
Microsoft Edge
_____
Microsoft Edge is the faster, safer browser built for Windows 10. It's optimized for the modern web, but is still compatible with legacy apps and sites.Always on security
The most significant new Windows 10 security feature involves a major improvement in authentication, based on biometric factors. Get essential business security features, built right into your device including BitLocker, which protects against loss of business information even when a device is lost or stolen.
____________________
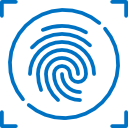
Windows Hello
Sign in to your Windows devices with Windows Hello in less than 2 seconds?3 times faster than a password. Facial or fingerprint recognition provides instant access, and you always have the option of retaining a PIN as a backup.
BitLocker
Keep your files safe with BitLocker, and get the same security on removable storage devices with BitLocker to Go. Windows 10 lets you create your own private app section in the Windows Store for convenient access to company applications


Block malicious software
To block malicious software after the boot process is complete, Windows 10 includes two signature features that will be new to any organization that is migrating directly from Windows 7: Windows Defender and Windows SmartScreen
Microsoft Windows 10 Pro - 1 License
While geared toward business customers, Windows 10 Professional is a good operating system choice for home users with powerful computer systems. My Choice Software makes it easy for you to install Windows 10 Professional with this 1 License version that will arrive quickly via electronic delivery. The design of Windows 10 takes the best features from the two prior operating systems, Windows 7 and Windows 8, and incorporates them into an operating system that works similarly well on PCs and tablets. This professional version of Windows 10 has many features that are not found on the Home version, including enterprise data protection, BitLocker and trusted boot for security, remote desktop, domain join and enterprise mode Internet Explorer for business use and the ability to join Azure Active Directory with a single sign-on to cloud-hosted applications. After purchasing the Windows 10 Pro key, you will receive one license activation code and a link to Microsoft's Media Creation Tool for Windows 10 Professional. Buy Windows 10 Pro now and enjoy the latest productivity solutions and a range of services designed to provide a seamless customer experience. Here are just a few of the industry-leading features that the Windows 10 Pro OS can offer:
Expanded Start menu: The familiar Start menu is back, providing quick, one-click access to the functions and files that people use most, and also a new space to personalize with favorite apps, programs, people, and websites. Select any app or program you want in order to always have it one click away.
Apps that run in Windows: Apps from the Windows Store now open in the same format that desktop programs do. They can be resized and moved around, and they have title bars at the top, allowing users to maximize, minimize, and close with a click.
Snap enhancements: Have up to four apps snapped on the same screen with a new quadrant layout. Windows will also show other apps and programs running for additional snapping, and even make smart suggestions about filling available screen space with other open apps.
New task view button: The new task view button on the task-bar enables one view for all open apps and files, allowing for quick switching and one-touch access to any desktops the user creates.
Multiple desktops: Instead of too many apps and files overlapping on a single desktop, it's easy to create, and switch between, distinct desktops for different purposes and projects, whether for work, personal use, or both.
Fulfilled within 30 minutes during normal business hours. When you buy Windows 10 Professional, You will receive (1) license activation code and a link to Microsoft's Media Creation Tool for Windows 10 Professional.