Windows 11 Download for Free - 2024 Latest Version
After releasing Windows 10 in 2015, Microsoft announced that it would be the last version of the popular operating system. Well, times change, and whether it was because of the technical advances or competition from Chrome OS, Microsoft released Windows 11 at the beginning of October. The design of Windows 11 will look familiar to anyone familiar with Chromebook, as it seems to have had a significant impact on the development team.
Don’t be fooled by the design, Windows 11 behaves and functions the same as Windows 10. The big improvement is with the features and compatibility with Android apps, quick access to Microsoft Teams, improved gaming, XBOX integration, and more.
There are also some visual upgrades to the interface that will make it more comfortable to use. This includes rounded corners instead of sharp edges, updated icons, and a nicer settings menu.
Follow the instructions below and I’ll show you how to get your free Windows 11 upgrade.
How to Download Your Windows 11 Upgrade for Free for PC
Microsoft has recently released Windows 11 as a free upgrade for everyone who has Windows 10. If you’re still using an older version of Windows, you will first need to upgrade to Windows 10, and then you’ll qualify for the Windows 11 upgrade. There are two ways to download Windows 11.
- Upgrade through your current Windows 10 OS: Open the Start Menu and click on the Settings button. Open the Update and Security section, and you’ll see the option to upgrade Windows. Click on the Download and Install button, and the installation will automatically begin.
- Download the exe file: If you don’t have the option to upgrade directly through Windows, Click on the Download button on the sidebar. This will open to the official Windows download page. Click the Download Now button, and the file will download on your computer.
Before Windows installs the upgrade, it will run a quick diagnostic test on your computer to ensure that it’s compatible with the requirements. If your computer can’t handle the upgrade, the installation will terminate, and you can continue using Windows 10. If your computer is compatible, follow the on-screen instruction, and the upgrade will install on your computer.
Windows 11 is a large file, and it can take up to a few hours to install on your computer. Therefore, don’t start the installation when you need the computer, but rather run it overnight or when you’re leaving the office.
Technical Requirements for Windows 11
Not every computer can handle the Windows upgrade. I like that Microsoft has a PC Health Check tool, which you can download on the same page where you download the exe file.
It scans your computer, and it will let you know if your computer has the resources to handle Windows 11.
- CPU: At least 1 GHz
- RAM: 4 GB
- Storage: 64 GB
- Trusted Platform Module (TPM): 2.0
- Firmware: Must support Secure Boot, UEFI
What’s New in Windows 11
The first thing that jumped out at me when I started using Windows 11 is that the start menu and program icons have moved from the left-hand corner where they’ve been for as long as I can remember.
Now, they sit right in the center of the menu bar on the bottom of the screen. I don’t think this will impact the usability or increase performance, but rather Microsoft wanted to make a visual statement that we’re entering a new era. If you don’t like the location, you can go to the settings and align it to the left.
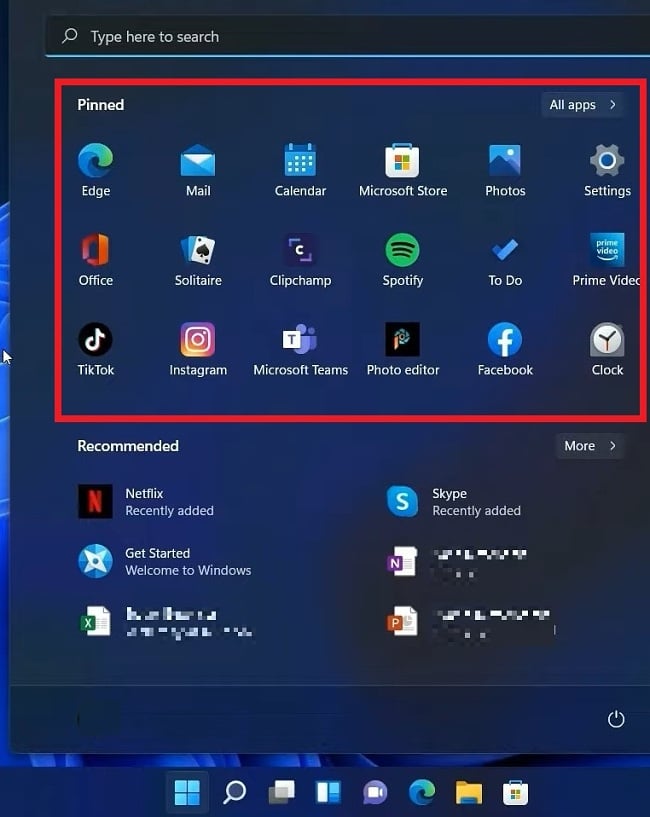
Updated Notification and Quick Setting Design
This update is likely to evoke a range of opinions. Individuals familiar with the Windows 10 Action Center, where clicking on each icon opened a dedicated window containing settings solely for that function, might find this update less to their preference. The problem is instead of different windows for each function, All functions are grouped together. For example, if you click on the Wi-Fi button, you’ll see all available networks, and if you click on the Volume icon, you can adjust the levels on your computer. With Windows 11, everything has been broken up into two panels.
The default icons on the tray are Wi-Fi, Battery, and Speaker, and when you click on any of them, a Quick Setting menu will open.
Here you can choose a Wi-Fi network, put your computer in Airplane Mode, turn on battery saver, adjust the volume, screen brightness, and turn on the Focus Assist feature. You can add different icons to this section, such as Connect for when using external monitors, Keyboard layout and languages, make your device discoverable, Night Light, and project your screen turn on sharing. A Pencil icon lets you customize what buttons appear, with a choice of Connect (for external displays and audio), Keyboard layout, Nearby sharing (like AirDrop for PCs), Night light, and Project.
Improved Widget Compatibility
While widgets were first introduced in Windows 7, they were quickly discontinued – until now. After seeing the popularity of widgets on mobile devices and Macs, Windows 11 has reinstated them, even adding a default icon on the menu bar for quick access. A widget is a part of an app that is always open on your screen, or in this case, stored in the Widget section. It makes it easier to access the app and see the important information you want without having to open the app. Some popular examples are sports widgets that show the scores of your favorite teams, a To-Do list, weather, calendar, notifications, and more. If you use the Microsoft Family Control to monitor your kid’s internet time, you can get a good overview of their online activity through the widget.
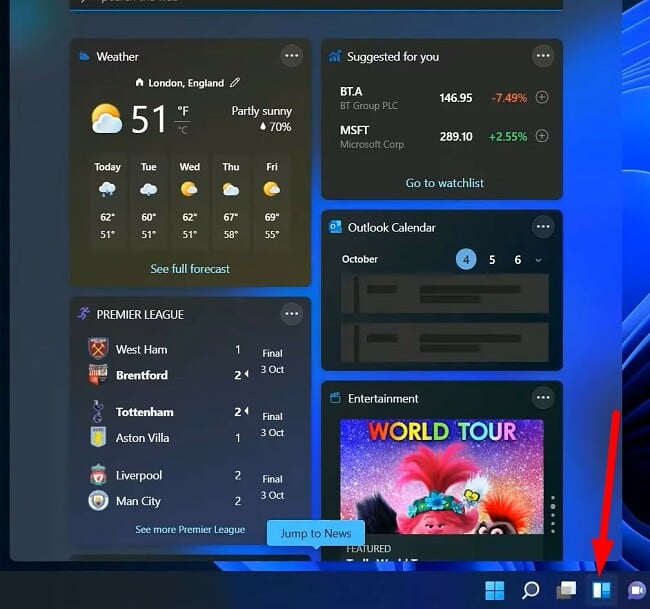
Click the Widget button to open the panel showing all your widgets
While it comes with several default Microsoft program widgets, like Outlook calendar, OneDrive, and a news feed, you can customize the widgets and stories you want to see and add third-party widgets as well.
Improved Productivity with Layouts and Multitasking Upgrades
One of the nice features that Windows has is the ability to organize app windows on your screen. With Windows 10, if you drag a window all to the right or left, it will take over half the screen, and you can create a split-screen view, which is nice if you’re working on two pages at the same time. Now, with the Windows 11 upgrade, you have a little more control in arranging your open websites and apps through the Snap Layout option. Hover your mouse over the “Maximize Window” button on the top corner of the window, and a layout display will open. You can choose what type of layout you want, whether it’s just split-screen or if you want to have four separate windows open at once. Windows will take your screen size into account, showing the different layout options to choose from.
I like the changes that Windows made to the virtual desktop feature. It’s a great tool for separating work and personal use or between parents and kids so that you can maintain some privacy. You can have multiple desktops open at once, and now Windows 11 allows you to have a different background for each desktop, making it easier to differentiate them. To scroll between different desktops, you can click on the Desktop icon on the taskbar or use the keyboard short TAB + Windows icon. If you’re using a touchscreen computer, you can use a four-finger swipe to move between desktops.
Better Integration with Microsoft Teams
Microsoft Teams soared in popularity, increasing its user base by seven times, largely thanks to the Covid-19 pandemic. With that in mind, Windows added its chat and video conferencing app to the center of the taskbar. When you click on the Teams icon the first time, you’ll need to sign in and allow Microsoft to access your account and contacts. Then, you’ll see a list of your contacts, and you’re just a click away from chatting or inviting someone to a video chat. If your contact isn’t using Teams, they’ll receive an invitation to join for free. You can also add Teams widgets to the widget section and quick links to get into other Teams apps and programs.
A Whole New Gaming Experience
Microsoft is looking to win the gaming battles, and Windows 11 goes a long way to helping them achieve this goal. They added two gaming components that gamers are sure to enjoy:
- Improved game selection
Microsoft included an XBOX app with this update. Now, players who have the XBOX Game Pass can access their games and play on their PC and XBOX. This includes the Halo collection, Marvel’s Avengers, Forza, Sea of Thieves, and hundreds of other games. Gamers can also access Microsoft’s streaming game platform, XBOX Cloud Gaming. - Better tech
A gaming experience can be significantly hampered by issues such as inconsistent performance, subpar graphics, and sluggish game loading. However, Windows 11 has effectively addressed these concerns through the inclusion of Auto HDR and DirectStorage. It is worth noting that Auto HDR was initially introduced in the Xbox Series X console. It helps games that weren’t developed with HDR attributes to have improved lighting, brightness, and contrast so they appear to have an HDR look for a higher quality gaming experience. DirectStorage is a part of the Xbox Velocity Architecture. It’s designed to allow the game to skip over the CPU so that the graphics memory can load directly. This allows the game to load even faster and avoid frustrating slowdowns due to an overburdened CPU.
Windows 11 also added Dynamic Refresh, which increases your laptop’s battery life by reducing the screen’s high refresh rate.
It also beefed up the security of the OS by requiring users to have TPM 2,0 and Secure Boot. These can help prevent DDoS attacks and plug vulnerabilities when gaming.
Stay Focused and On Task
One of the features that will probably help me the most is Focus Assist. It is integrated into the clock app and is designed to help increase productivity with the task completion settings. You can create a list of things to do, along with a timer, to help keep you focused on the task at hand. When you complete a task, just mark it off and move on with your day. Focus Assist has Spotify integration, so you can set the appropriate music or background noise for each task.
Coming Soon: Android Compatibility
One of the most exciting features that Microsoft revealed is that it will be compatible with most Android apps. This means that instead of downloading APK files through an emulator program like Bluestacks, you will be able to download, install, and run the apps directly from your computer. Microsoft, Google, and Amazon have all agreed to work together to make this possible – instead of downloading apps from the Google Play Store, you will need to download them from the Amazon Appstore, which will run through the Microsoft Windows Store. While it sounds a bit confusing and quite the workaround, Microsoft’s Chief Product Officer Panos Panay promises that downloading and using Android apps will be “Smooth and Easy.”
FAQ
There are three possible reasons that you can’t download Windows 11:
- Windows announced that they are slowly rolling out the upgrade as they continue to make improvements and adjustments, so it could be that it’s just not available where you are.
- Your computer doesn’t meet the requirements needed to run Windows 11.
- You have a pirated or unauthorized version of Windows 10, and therefore don’t qualify for a free upgrade.